Framework of ITI-GEN
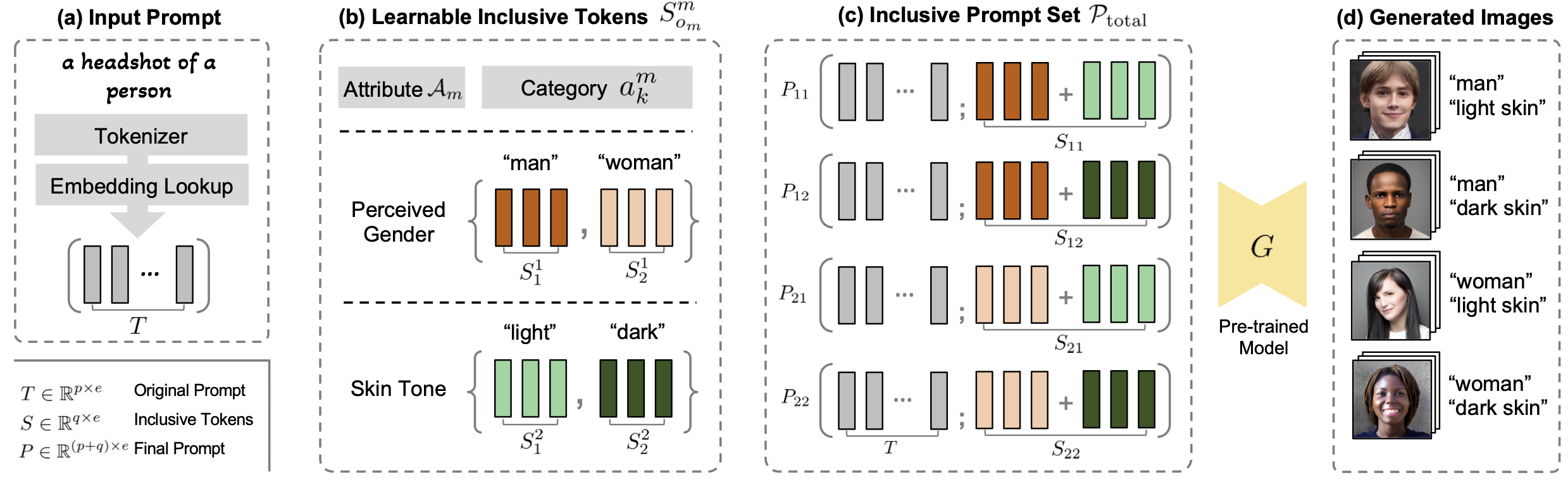
Illustration of Inclusive Text-to-Image Generation with the example of two binary attributes: perceived gender and skin tone. (a) Given an input prompt, (b) ITI-GEN learns discriminative token embeddings to represent each category of every target attribute. (c) By injecting the learned tokens after the original input prompt, ITI-GEN synthesizes an inclusive prompt set that can be used to (d) sample equal (or controllable) numbers of images for any category combination. Further, our framework can be easily extended to multi-category multi-attribute scenarios of inclusive text-to-image generation. Note that, in practice, multi-category skin tones beyond {“light”, “dark”} as in this example may be challenging to specify with language.